Prompt Chaining
Introduction to Prompt Chaining
LLMμ μμ μ±κ³Ό μ±λ₯μ κ°μ νκΈ° μν΄ μ€μν ν둬ννΈ μμ§λμ΄λ§ κΈ°λ² μ€ νλλ μμ μ νμ μμ μΌλ‘ λΆν νλ κ²μ λλ€. μ΄λ¬ν νμ μμ μ΄ μλ³λλ©΄ LLMμ νμ μμ μ λν ν둬ννΈκ° νμλκ³ κ·Έ μλ΅μ΄ λ€λ₯Έ ν둬ννΈμ μ λ ₯μΌλ‘ μ¬μ©λ©λλ€. ν둬ννΈ μ°μλΌλ κ°λ μΌλ‘ μμ μ νμ μμ μΌλ‘ λΆν νμ¬ ν둬ννΈ μμ μ μ°μλ₯Ό λ§λλ κ²μ ν둬ννΈ μ²΄μ΄λ μ΄λΌκ³ ν©λλ€.
ν둬ννΈ μ²΄μ΄λμ λ§€μ° μμΈν ν둬ννΈλ‘ ν둬ννΈλ₯Ό λ³΄λΌ κ²½μ° LLMμ΄ μ²λ¦¬νκΈ° μ΄λ €μΈ μ μλ 볡μ‘ν μμ μ μννλ λ° μ μ©ν©λλ€. ν둬ννΈ μ²΄μ΄λμμ ν둬ννΈλ μ΅μ’ μνλ μνμ λλ¬νκΈ° μ μ μμ±λ μλ΅μ λν΄ λ³ν λλ μΆκ° νλ‘μΈμ€λ₯Ό μνν©λλ€.
ν둬ννΈ μ²΄μ΄λμ λ λμ μ±λ₯μ λ¬μ±νλ κ² μΈμλ LLM μ ν리μΌμ΄μ μ ν¬λͺ μ±μ λμ΄κ³ μ μ΄ κ°λ₯μ± λ° μμ μ±μ λμ΄λ λ° λμμ΄ λ©λλ€. μ¦, λͺ¨λΈ μλ΅μ λ¬Έμ λ₯Ό ν¨μ¬ μ½κ² λλ²κ·Ένκ³ κ°μ μ΄ νμν μ¬λ¬ λ¨κ³μ μ±λ₯μ λΆμνκ³ κ°μ ν μ μμ΅λλ€.
ν둬ννΈ μ²΄μ΄λμ LLM κΈ°λ° λνν μ΄μμ€ν΄νΈλ₯Ό ꡬμΆνκ³ μ ν리μΌμ΄μ μ κ°μΈν λ° μ¬μ©μ κ²½νμ κ°μ ν λ νΉν μ μ©ν©λλ€.
ν둬ννΈ μ²΄μ΄λ μ¬μ© μ¬λ‘
λ¬Έμ QAλ₯Ό μν ν둬ννΈ μ²΄μ΄λ
ν둬ννΈ μ²΄μΈμ΄λμ μ¬λ¬ μμ μ΄λ λ³νμ μλ°ν μ μλ λ€μν μλ리μ€μμ μ¬μ©ν μ μμ΅λλ€. μλ₯Ό λ€μ΄, LLMμ μΌλ°μ μΈ μ¬μ© μ¬λ‘ μ€ νλλ ν° ν μ€νΈ λ¬Έμμ λν μ§λ¬Έμ λ΅νλ κ²μ λλ€. 첫 λ²μ§Έ ν둬ννΈλ μ§λ¬Έμ λ΅νκΈ° μν΄ κ΄λ ¨ μΈμ©λ¬Έμ μΆμΆνκ³ λ λ²μ§Έ ν둬ννΈλ μ£Όμ΄μ§ μ§λ¬Έμ λ΅νκΈ° μν΄ μΈμ©λ¬Έκ³Ό μλ³Έ λ¬Έμλ₯Ό μ λ ₯μΌλ‘ λ°λ λ κ°μ μλ‘ λ€λ₯Έ ν둬ννΈλ₯Ό λμμΈνλ©΄ λμμ΄ λ©λλ€. μ¦, λ¬Έμκ° μ£Όμ΄μ‘μ λ μ§λ¬Έμ λ΅νλ μμ μ μννκΈ° μν΄ μλ‘ λ€λ₯Έ λ κ°μ ν둬ννΈλ₯Ό λ§λ€κ² λ©λλ€.
μλμ 첫 λ²μ§Έ ν둬ννΈλ μ§λ¬Έμ΄ μ£Όμ΄μ§ λ¬Έμμμ κ΄λ ¨ μΈμ©λ¬Έμ μΆμΆν©λλ€. κ°λ¨νκ² νκΈ° μν΄ {{document}}
λ¬Έμμ λν μ리 νμμλ₯Ό μΆκ°νμ΅λλ€. ν둬ννΈλ₯Ό ν
μ€νΈνλ €λ©΄ ν둬ννΈ μμ§λμ΄λ§ (opens in a new tab)μ λν μ΄ νμ΄μ§μ κ°μ Wikipedia λ¬Έμλ₯Ό 볡μ¬νμ¬ λΆμ¬λ£μ μ μμ΅λλ€. μ΄ μμ
μλ λ ν° μ»¨ν
μ€νΈκ° μ¬μ©λκΈ° λλ¬Έμ OpenAIμ gpt-4-1106-preview
λͺ¨λΈμ μ¬μ©νκ³ μμ΅λλ€. μ΄ ν둬ννΈλ Claudeμ κ°μ λ€λ₯Έ κΈ΄ 컨ν
μ€νΈμ LLMκ³Ό ν¨κ» μ¬μ©ν μ μμ΅λλ€.
Prompt 1:
You are a helpful assistant. Your task is to help answer a question given a document. The first step is to extract quotes relevant to the question from the document, delimited by ####. Please output the list of quotes using <quotes></quotes>. Respond with "No relevant quotes found!" if no relevant quotes were found.
####
{{document}}
####
λ€μμ user
μν μ μ¬μ©νμ¬ μ λ¬λλ μ§λ¬Έμ ν¬ν¨ν μ 체 ν둬ννΈμ μ€ν¬λ¦°μ·μ
λλ€.
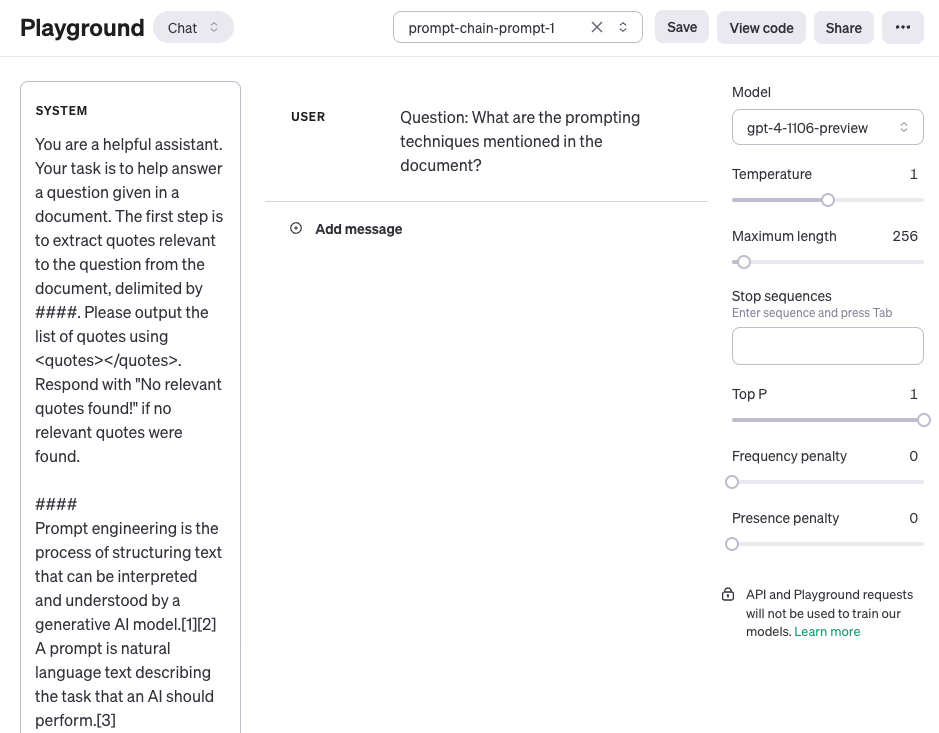
Output of Prompt 1:
<quotes>
- Chain-of-thought (CoT) prompting[27]
- Generated knowledge prompting[37]
- Least-to-most prompting[38]
- Self-consistency decoding[39]
- Complexity-based prompting[41]
- Self-refine[42]
- Tree-of-thought prompting[43]
- Maieutic prompting[45]
- Directional-stimulus prompting[46]
- Textual inversion and embeddings[59]
- Using gradient descent to search for prompts[61][62][63][64]
- Prompt injection[65][66][67]
</quotes>
μ΄μ 첫 λ²μ§Έ ν둬ννΈμμ λ°νλ λ°μ΄νλ₯Ό μλ λ λ²μ§Έ ν둬ννΈμ μ λ ₯μΌλ‘ μ¬μ©ν μ μμ΅λλ€. λ°μ΄νλ₯Ό μ‘°κΈ λ μ 리ν μ μμ΅λλ€. μ¦, μΈμ©μ μ κ±°ν μ μμ΅λλ€. μ΄λ¬ν μΈμ©λ¬Έμ μ κ±°νκ±°λ 체μΈμ λ€λ₯Έ ν둬ννΈμ μΌλΆλ‘ νμ©ν μ μμ§λ§ μ§κΈμ 무μν©λλ€. κ·Έλ¬λ©΄ λ λ²μ§Έ ν둬ννΈλ ν둬ννΈ 1μμ μΆμΆν κ΄λ ¨ μΈμ©λ¬Έμ κ°μ Έμ λ¬Έμμ μΆμΆλ μΈμ©λ¬Έμ λ°νμΌλ‘ μ§λ¬Έμ λν μ μ©ν λ΅λ³μ μ€λΉν©λλ€. λ λ²μ§Έ ν둬ννΈλ λ€μκ³Ό κ°μ μ μμ΅λλ€:
Prompt 2:
Given a set of relevant quotes (delimited by <quotes></quotes>) extracted from a document and the original document (delimited by ####), please compose an answer to the question. Ensure that the answer is accurate, has a friendly tone, and sounds helpful.
####
{{document}}
####
<quotes>
- Chain-of-thought (CoT) prompting[27]
- Generated knowledge prompting[37]
- Least-to-most prompting[38]
- Self-consistency decoding[39]
- Complexity-based prompting[41]
- Self-refine[42]
- Tree-of-thought prompting[43]
- Maieutic prompting[45]
- Directional-stimulus prompting[46]
- Textual inversion and embeddings[59]
- Using gradient descent to search for prompts[61][62][63][64]
- Prompt injection[65][66][67]
</quotes>
Output of Prompt 2:
The prompting techniques mentioned in the document include:
1. Chain-of-thought (CoT) prompting[27]
2. Generated knowledge prompting[37]
3. Least-to-most prompting[38]
4. Self-consistency decoding[39]
5. Complexity-based prompting[41]
6. Self-refine[42]
7. Tree-of-thought prompting[43]
8. Maieutic prompting[45]
9. Directional-stimulus prompting[46]
10. Textual inversion and embeddings[59]
11. Using gradient descent to search for prompts[61][62][63][64]
12. Prompt injection[65][66][67]
Each of these techniques employs unique strategies to enhance or specify the interactions with large language models to produce the desired outcomes.
보μλ€μνΌ ν둬ννΈ μ²΄μΈμ λ¨μννκ³ μμ±νλ κ²μ μλ΅μ΄ μ¬λ¬ κ°μ§ μμ μ΄λ λ³νμ κ±°μ³μΌ νλ κ²½μ° μ μ©ν ν둬ννΈ μ κ·Ό λ°©μμ λλ€. μ°μ΅ μΌμ μ ν리μΌμ΄μ μ¬μ©μμκ² μ΅μ’ μλ΅μΌλ‘ 보λ΄κΈ° μ μ μλ΅μμ μΈμ©λ¬Έ(μ: [27])μ μ κ±°νλ ν둬ννΈλ₯Ό μμ λ‘κ² λμμΈν΄ 보μΈμ.
λν μ΄ λ¬Έμ (opens in a new tab)μμ Claude LLMμ νμ©ν ν둬ννΈ μ²΄μ΄λμ λ λ§μ μμλ₯Ό μ°Ύμ μ μμ΅λλ€. μ΄ μμ λ μ΄λ¬ν μμ μμ μκ°μ λ°μ μμ ν κ²μ λλ€.